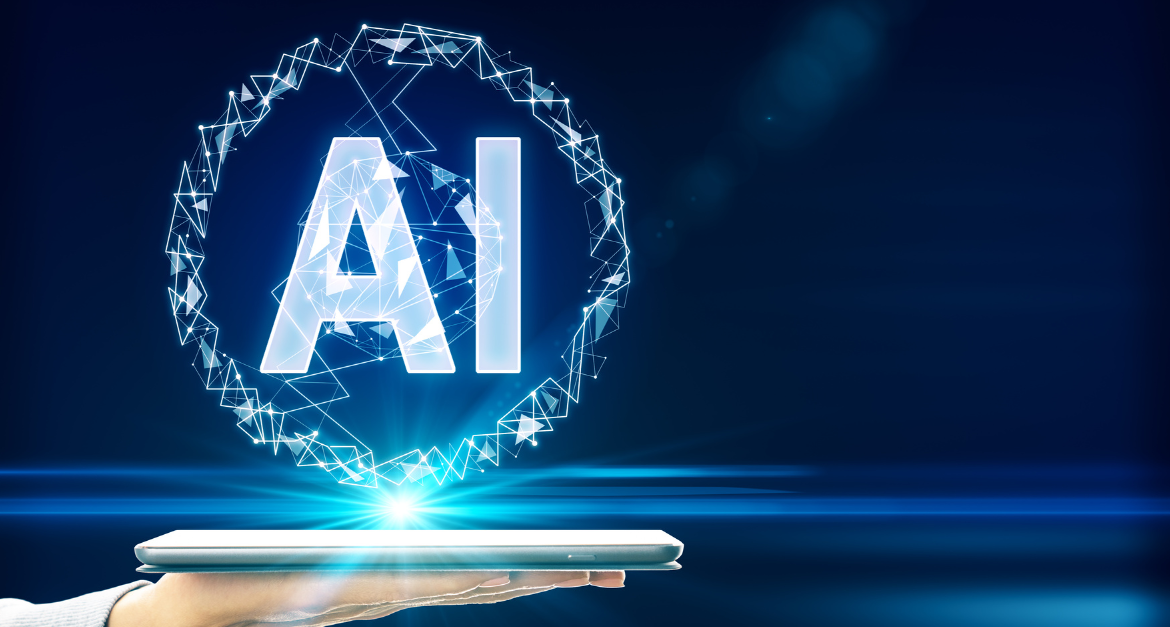
The Transformative Power of AI in Customer Experience: Enhancing Organizations’ Efficiency and ROI
Artificial Intelligence (AI) has quickly emerged as a pivotal technology for organizations aiming to revolutionize their customer experience. With its ability to streamline processes, personalize interactions, and enable predictive analytics, AI empowers companies to deliver exceptional customer service. In this article, we will explore what AI in customer experience entails, how organizations can harness this technology, and its potential Return on Investment (ROI) through real-world examples.
What is AI in Customer Experience?
AI in customer experience refers to the integration of artificial intelligence technology, such as machine learning, natural language processing, and chatbots, to enhance customer interactions, satisfaction, and operational efficiency. By automating processes (see also our process manager) and analyzing vast amounts of customer data, AI enables companies to provide personalized experiences at scale, improving both customer loyalty and business outcomes.
Leveraging AI for Enhanced Customer Experience:
Personalized Recommendations: AI algorithms can analyze customer behavior, preferences, and purchase history to offer personalized product recommendations, enhancing the shopping experience and increasing cross-selling and upselling opportunities.
Chatbots and Virtual Assistants: AI-powered chatbots and virtual assistants can provide instant and accurate responses to customer inquiries, 24/7. They can handle routine queries, offer product information, and help with basic troubleshooting, improving customer satisfaction and reducing response times. Using natural language queries in customer experience enables businesses to provide personalized and seamless interactions, enhancing the overall user experience. Chatbots and voice assistants can quickly and accurately interpret customer queries, understand their intents, and offer relevant responses or actions.
By incorporating natural language processing capabilities into customer experience platforms, businesses can foster better engagement and satisfaction, as customers can access information or receive support in a more conversational and user-friendly way.
Sentiment Analysis: AI algorithms can analyze customer feedback, reviews, and social media posts to determine sentiment and gauge customer satisfaction levels. This helps companies identify areas of improvement, address concerns promptly, and tailor their offerings to meet customer expectations.
Voice Recognition and Natural Language Processing: AI-powered voice recognition and natural language processing technologies enable companies to provide voice-activated customer service and support. Customers can interact with devices or applications using voice commands, making interactions more convenient and intuitive.
Predictive Customer Analytics: AI can analyze large volumes of customer data to identify patterns and predict future behavior, such as customer churn or purchase likelihood. This helps companies proactively address customer needs and tailor their marketing campaigns and offers more effectively.
Virtual Reality (VR) and Augmented Reality (AR): AI can be used in conjunction with VR and AR technologies to offer immersive and interactive experiences. For instance, customers can use VR to try on clothes virtually or use AR to visualize how furniture might look in their homes, enhancing the pre-purchase experience.
Self-Service and Automation: AI-powered self-service platforms enable customers to find answers to their queries or access product information independently. Automation of routine tasks, such as order tracking or appointment scheduling, enhances efficiency and reduces customer effort.
Voice of the Customer Analysis: AI can analyze customer feedback across various channels to identify trends, patterns, and common issues. This helps companies understand the voice of the customer, prioritize improvements, and make data-driven decisions to enhance the overall customer experience.
Intelligent Routing and Prioritization: AI algorithms can automatically route customer inquiries to the most suitable customer support representative based on expertise or customer segmentation. This ensures that customers receive personalized and efficient service, reducing wait times and improving resolution rates.
Some real-world examples of applying Ai in the area of CX
Lloyds Banking Group:
Lloyds Banking Group, one of the largest financial institutions in the UK, has implemented AI technology to improve its customer experience. The bank uses virtual assistants powered by AI to handle simple customer queries across various channels, including web chat and mobile apps. These assistants provide customers with instant responses, saving time and enhancing satisfaction. By leveraging AI for customer support, Lloyds Banking Group has reduced waiting times, improved efficiency, and increased customer engagement.
Deutsche Bahn:
Deutsche Bahn, the German railway company, has integrated AI technology to enhance customer experience in its customer service operations. The company has developed an AI-powered chatbot named “DB Reise Bot” that assists travelers with real-time information, ticket bookings, and personalized recommendations. The chatbot leverages natural language processing capabilities to understand and respond to customer queries in a conversational manner. By utilizing AI in its customer service, Deutsche Bahn has improved the speed and accuracy of information provided to customers, leading to higher satisfaction levels and increased efficiency.
These examples showcase how organizations in different industries are leveraging AI to enhance their customer experience, address customer queries promptly, and deliver personalized services, irrespective of geographical location.
Where is the ROI of applying AI in Customer Experience:
Cost Reduction and Productivity Increase: AI-driven automation optimizes processes, reducing the need for manual intervention and saving costs associated with human resources. Additionally, AI enhances efficiency by directing customer queries to the right channels, minimizing handling times, and reducing overall operational costs.
Increased Customer Satisfaction and Loyalty: Personalization, proactive issue resolution, and AI-enabled support contribute to higher customer satisfaction and loyalty. Satisfied customers are more likely to repeat purchases, refer others, and become brand advocates, driving revenue growth.
Data sensitivity
Ensuring the security of AI systems is crucial, not only to protect sensitive customer data but also to comply with data privacy regulations, such as the General Data Protection Regulation (GDPR) in the EU. The consequences of AI systems breaches could be severe, leading to reputational damage, legal and regulatory implications, and financial losses.
Encryption is a vital security measure that can help companies protect customer data from unauthorized access, a core tenet of GDPR. Encryption works by masking the data as it travels from one point to another, making it unreadable to anyone who does not have the key to decrypt it. Access controls limit access to AI systems and data to authorized personnel only, reducing the risk of data breaches and ensuring GDPR compliance.
Data anonymization is also an important security measure, particularly for companies that use customer data for AI training purposes. Anonymization removes personally identifiable information from data, further reducing the risk of data breaches and ensuring GDPR compliance.
Implementing robust security measures is critical to protect AI systems and the data they process, comply with data privacy regulations such as GDPR, and safeguard customer data. Encryption, access controls, and data anonymization are just a few examples of the many security measures that companies should consider when implementing AI solutions. By prioritizing AI security and complying with applicable data privacy regulations such as GDPR, companies can mitigate the risks associated with data breaches, protect customer data, and build customer trust.
Out-of-the-Box AI solutions
Out-of-the-box AI solutions are often referred to as a “black box” because they offer limited visibility and control over how and where data is processed, stored, and transformed. When implementing such solutions, businesses typically rely on pre-built AI models, algorithms, or services provided by external vendors.
With these pre-packaged solutions, organizations have minimal control or knowledge about the inner workings of the AI system. They do not have direct control over the infrastructure, data processing techniques, or storage methods employed by the solution. As a result, the intricacies of data processing and transformations remain opaque, making it challenging to understand the specific algorithms and logic used to process and transform the data.
Furthermore, the actual location where data is processed and stored may also be unknown or controlled by the vendor providing the out-of-the-box AI solution. This lack of transparency can raise concerns regarding data privacy, security, and compliance, especially when dealing with sensitive or regulated data.
While these out-of-the-box solutions offer convenience and ease of implementation, they often sacrifice the ability to tailor the AI system to specific organizational requirements. The lack of control over data processing, storage, and transformations can hinder organizations’ ability to customize the AI solution according to their unique needs or comply with specific data governance policies.
To address these limitations, organizations may opt for more customizable AI solutions or consider building their own AI capabilities in-house. This allows for greater control over data processing, storage, and transformations, ensuring compliance, data security, and alignment with organizational requirements.
Choosing out-of-the-box AI solutions means accepting a trade-off between convenience and control. While they offer quick deployment and ease of use, they can limit visibility and control over data. Businesses should carefully evaluate their specific needs and prioritize control and transparency when deciding between out-of-the-box solutions
Data architecture & considerations that can scale resources based on AI workload
Computational power requirements vary depending on the complexity and scale of AI workloads. By designing an architecture that can scale resources up or down, companies can ensure that they have sufficient computational power to handle the processing needs of AI algorithms. This scalability allows organizations to effectively meet fluctuating demands, such as during peak customer engagement periods or when running resource-intensive AI models.
Scaling resources based on workload demands improves also cost-efficiency. By dynamically allocating resources as needed, companies can avoid overprovisioning, which can result in unnecessary expenses. On the other hand, scaling down resources during off-peak periods helps optimize resource utilization and reduce costs.
In addition to computational power, strong management in terms of data governance and data management strategy is paramount. AI systems rely on high-quality and well-organized data for training and inference. As AI systems scale, data governance becomes increasingly important to ensure data quality, proper data access controls, and compliance with data privacy regulations.
Implementing a robust data management strategy is also crucial to handle the increasing volumes of data generated by AI systems. This includes data storage, data indexing, and data retrieval mechanisms that can efficiently handle large datasets. By designing a scalable architecture with provisions for effective data governance and management, companies can ensure the availability, integrity, and security of the data that fuels their AI systems.
The importance of designing a scalable architecture that can cater to AI workload demands cannot be overstated. It enables companies to efficiently handle varying workloads, optimize costs, maintain data quality, ensure compliance, and derive maximum value from their AI investments.
Conclusion
AI is revolutionizing the customer experience landscape, empowering organizations to deliver personalized, efficient, and effective interactions. From personalized recommendations to AI-enabled chatbots, companies can leverage AI to enhance customer satisfaction while reducing costs. As organizations continue to prioritize exceptional customer experiences, AI will play a crucial role in their success but will be challenged by the machine power and data management processes that comes with it to maximize the power of this technology.